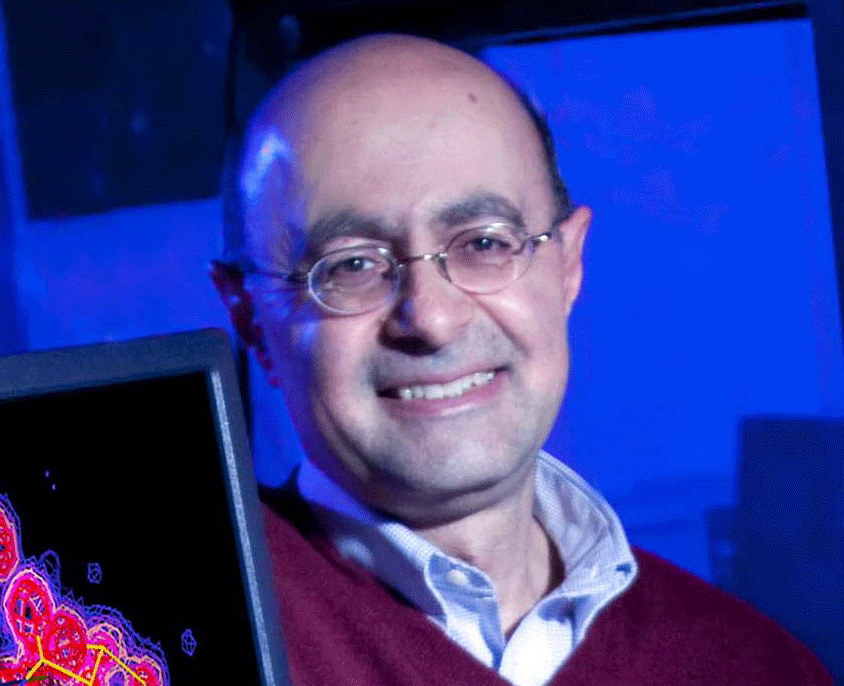
Popular Articles
- Earliest molecular events of vision revealed
- Crystal structure of CO-bound cytochrome c oxidase determined by serial femtosecond X-ray crystallography at room temperature
- XFEL Pulses Demonstrate How Plants Perceive Light
- NSF awards $22.5 million to capture biology at the atomic level using X-ray lasers
- BioXFEL Postdoctoral Fellowship Award
Archived Articles
- Details
- Tuesday, 23 June 2020
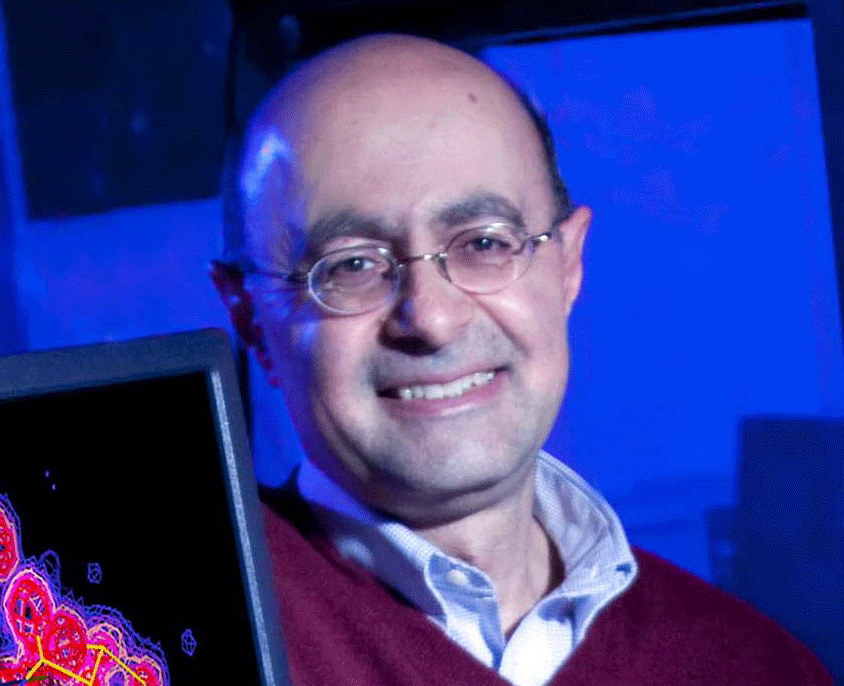
Knowing the gestational age of a baby is key for optimal pregnancy management and in order to know whether birth is premature or not. This is important for pregnant women and their babies, as preterm birth is a major global health challenge and the leading cause of death in children under 5 years old. However, determining the gestational age accurately is not easy: for most women the date of conception is only roughly known, and even babies conceived at the same time grow at different rates. The accuracy of gestational age is made more difficult still when women seek antenatal care late, as is commonly the case in low income settings. All this means that gestational age determination is associated with a wide prediction interval: for example, usual ways of estimation at 26 weeks are accurate to only +/- 14 days.
Now, an international group of researchers led by data scientists at the University of Wisconsin-Milwaukee have applied a machine learning technique they developed to substantially reduce the uncertainty in gestational age estimation; in the example above, the accuracy is +/- 3 days evaluated by predicting intervals between visits, and is possible to achieve in 75% of women with just a single ultrasound. The paper was published today in The Lancet Digital Health.
“Applying this technique to neonatal health has the potential to improve management of individual pregnancies, and boost population-level health”, said Abbas Ourmazd, UWM Distinguished Professor of physics and a corresponding author of the paper. In addition it will allow for more accurate statistics on premature birth, a key measure of a population’s general health and nutritional status.
The machine learning algorithm, created by Senior Scientist Russell Fung and Abbas Ourmazd, together with Prof. Aris Papageorghiou and colleagues at the University of Oxford. The algorithm corrects for time uncertainties in past events by identifying correlations in the data that help fill in some of the missing pieces. “Compared to existing techniques, the new method results in a 3-5 fold reduction in uncertainty in determining gestational age ” said Aris Papageorghiou. “The system has been tested and works very well at 20-30 weeks of pregnancy. We are continuing our efforts to improve gestational age determination over a wider range.”
The researchers used data from a global study on the health of mothers and babies during pregnancy and through the children’s second year. The International Fetal and Newborn Growth Consortium for the 21st Century, coordinated by the team at the University of Oxford, involved a network of more than 300 researchers and clinicians from 27 institutions in 18 countries worldwide dedicated to improving perinatal health globally.
The machine learning algorithm was originally developed to make ultra-slow-motion movies of exploding molecules. First reported in the journal Nature in 2016 [https://www.nature.com/articles/nature17627/], the technique has numerous applications in science, said Ourmazd.
Upon publication of this paper, the new algorithm will be made available for research purposes free of charge through a web portal.
This study was supported by generous grants from the Bill & Melinda Gates Foundation to the Universities of Oxford and Wisconsin Milwaukee. The article contents are the sole responsibility of the authors, and may not necessarily represent the official views of the Gates Foundation or other agencies, which may have supported the studies used in the present study. The development of underlying techniques was supported by the US Department of Energy, Office of Science, Basic Energy Sciences under award DE-SC0002164 (underlying dynamical techniques), and by the US National Science Foundation under awards STC 1231306 (underlying data analytical techniques) and 1551489 (underlying analytical models). Aris T. Papageorghiou is supported by the National Institute for Health Research (NIHR) Oxford Biomedical Research Centre (BRC).